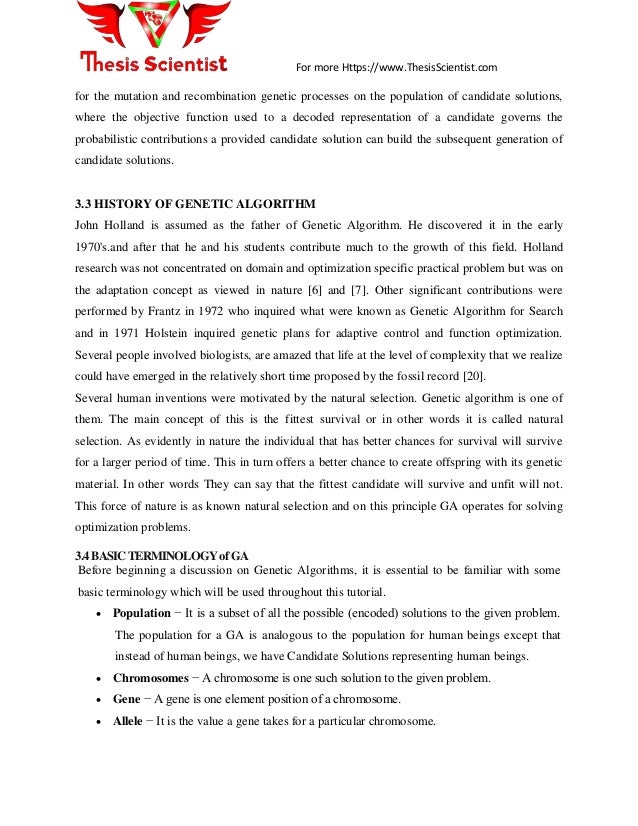
This is the first stable release of Apache Hadoop x line. It contains bug fixes, improvements and enhancements since Users are encouraged to read the overview of major changes since For details of bug fixes, improvements, and other enhancements since the previous release, please check release notes and changelog detail the changes since Ad hoc progress reviews: please email grs@blogger.com with the date you would like the review due and we will send you a link to the form; Change to Progress Committee Chair and/or independent members online form; Requests to postpone a progress milestone can be submitted via the online Extension to candidature or expected work submission Master of Engineering. The master of engineering (ME) degree is a two-year program of advanced courses with research leading to a master’s thesis. ME candidates must complete 16 courses (64 credits), including 8 research-oriented courses at the level, complete a thesis
Hopfield network - Wikipedia
A Hopfield network or Ising model of a neural network or Ising—Lenz—Little model is a form of recurrent artificial neural network and a type of spin glass system popularised by John Hopfield in [1] as described earlier by Little in [2] based on Ernst Ising 's work master thesis ad hoc networks Wilhelm Lenz on the Ising model.
Hopfield networks also provide a model for understanding human memory. Ising model of a neural network as a memory model is first proposed by William A.
Little in[2] which is acknowledged by Hopfield in his paper. The units in Hopfield nets are binary threshold units, i. Discrete Hopfield master thesis ad hoc networks describe relationships between binary firing or not-firing neurons 12. However, other literature might use units that take values of 0 and 1. The constraint that weights are symmetric guarantees that the energy function decreases monotonically while following the activation rules.
Hopfield also modeled neural nets for continuous values, in which the electric output of each neuron is not binary but some value between 0 and 1.
Updating one unit node in the graph simulating the artificial neuron in the Hopfield network is performed using the following rule:. The weight between two units has a powerful impact upon the values of the neurons. Thus, the values of neurons i and j will converge if the weight between them is positive. Similarly, they will diverge if the weight is negative.
Bruck shed light on the behavior of a neuron in the discrete Hopfield network when proving its convergence in his paper in Bruck shows [8] that neuron master thesis ad hoc networks changes its state if and only if it further decreases the following biased pseudo-cut. The discrete Hopfield network minimizes the following biased pseudo-cut [9] for the synaptic weight matrix of the Hopfield net.
For further details, see the recent paper. The discrete-time Hopfield Network always minimizes exactly the following pseudo-cut [8] [9]. The continuous-time Hopfield network always minimizes an upper bound to the following weighted cut [9]. where f. The complex Hopfield network, on the other hand, generally tends to minimize the so-called shadow-cut of the complex weight matrix of the net.
Hopfield nets have a scalar value associated with each state of the network, referred to as the "energy", E, of the network, where:. This quantity is called "energy" because it either decreases or stays the same upon network units being updated, master thesis ad hoc networks.
Furthermore, under repeated updating the network will eventually converge to a state which is a local minimum in the energy function which is considered to be a Lyapunov function. Note that this energy function belongs to a general class of models in physics under the name of Ising models ; these in turn are a special case of Markov networksmaster thesis ad hoc networks, since the associated probability measurethe Gibbs measuremaster thesis ad hoc networks, has the Markov property.
Hopfield and Tank presented the Hopfield network application in solving the classical traveling-salesman problem in Although including the optimization constraints into the synaptic weights in the best possible way is a challenging task, indeed many various difficult optimization problems with constraints in different disciplines have been converted to the Hopfield energy function: Associative memory systems, Analog-to-Digital conversion, job-shop scheduling problem, quadratic assignment and other related NP-complete problems, channel allocation problem in wireless networks, mobile ad-hoc network routing problem, image restoration, system identification, combinatorial optimization, etc, just to name a few.
Further details can be found in e. the paper. Initialization of the Hopfield networks is done by setting the values of the units to the desired start pattern. Repeated updates are then performed until the network converges to an attractor pattern. Convergence is generally assured, as Hopfield proved that the attractors of this nonlinear dynamical system are stable, not periodic or chaotic as in some other systems [ citation needed ].
Therefore, in the context of Hopfield networks, an attractor pattern is a final stable state, a pattern that cannot change any value within it under updating [ citation needed ].
Training a Hopfield net involves lowering the energy of states that the net should "remember". This allows the net to serve as a content addressable memory system, that is to say, the network will converge to a "remembered" state if it is given only part of the state. The net can be used to recover from a distorted input to the trained state that is most similar to that input.
This is called associative memory because it recovers memories on the basis of similarity. For example, if we train a Master thesis ad hoc networks net with five units so that the state 1, -1, 1, -1, 1 is an energy minimum, master thesis ad hoc networks, and we give the network the state 1, -1, -1, -1, 1 it will converge to 1, -1, 1, -1, 1.
Thus, the network is properly trained when the energy of states which the network should remember are local minima. Note that, in contrast to Perceptron training, master thesis ad hoc networks, the thresholds of the neurons are never updated.
There are various different learning rules that can be used to store information in the memory of the Hopfield network. It is desirable for a learning rule to have both of the following two properties:. These properties are desirable, since a learning rule satisfying them is more biologically plausible. For master thesis ad hoc networks, since the human brain is always learning new concepts, one can reason that human learning is incremental. A learning system that was not incremental would generally be trained only once, with a huge batch of training data.
The Hebbian Theory was introduced by Donald Hebb inin order to explain "associative learning", in which simultaneous activation of neuron cells leads to pronounced increases in synaptic strength between those cells. Neurons that fire out of sync, fail to link". The Hebbian rule is both local and incremental. The opposite happens if the bits corresponding to neurons i and j are different.
This rule was introduced by Amos Storkey in and is both local and incremental. Storkey also showed that a Hopfield network trained using this rule has a greater capacity than a corresponding network trained using the Hebbian rule. This learning rule is local, since the synapses take into account only neurons at their sides.
The rule makes use of more information from the patterns and weights than the generalized Hebbian rule, due to the effect of the local field. Patterns that the network uses for training called retrieval states become attractors of the system.
Repeated updates would eventually lead to convergence to one of the retrieval states. However, sometimes the network will converge to spurious patterns different from the training patterns. For each stored pattern x, the negation -x is also a spurious pattern. A spurious state can also be a linear combination of an odd number of retrieval states. Spurious patterns that have an master thesis ad hoc networks number of states cannot exist, since they might sum up to zero [15].
The Network capacity of the Hopfield network model is determined by neuron amounts and connections within a given network. Therefore, the number of memories that are able to be stored is dependent on neurons and connections. Furthermore, it was shown that the recall accuracy between vectors and nodes was 0.
Therefore, it is evident that many mistakes will occur if one tries to store a large number of vectors. When the Hopfield model does not recall the right pattern, it is possible that an intrusion has taken place, since semantically related items tend to confuse the individual, and recollection of the wrong pattern occurs.
Therefore, the Hopfield network model is shown to confuse one stored item with that of another upon retrieval. The Hopfield model accounts for associative memory through the incorporation of memory vectors. Memory vectors can be slightly used, and this would spark the retrieval of the most similar vector in the network. However, master thesis ad hoc networks, we will find out that due to this process, intrusions can occur. In associative memory for the Hopfield network, there are two types of operations: auto-association and hetero-association.
The first being when a vector is associated with itself, and the latter being when two different vectors are associated in storage. Furthermore, both types of operations are possible to store within a single memory matrix, but only if that given representation matrix is not one or the other of the operations, but rather the combination auto-associative and hetero-associative of the two.
It is important to note that Hopfield's network model utilizes the same learning rule as Hebb's learning rulewhich basically tried to show that learning occurs as a result of the strengthening of master thesis ad hoc networks weights by when activity is occurring. Rizzuto and Kahana were able to show that the neural network model can account for repetition on recall accuracy by incorporating a probabilistic-learning algorithm. During the retrieval process, no learning occurs.
As a result, the weights of the network remain fixed, showing that the model is able to switch from a learning stage to a recall stage. By adding contextual drift they were able to show the rapid forgetting that occurs in a Hopfield model during a cued-recall task. Master thesis ad hoc networks entire network contributes to the change in the activation of any single node. McCulloch and Pitts' dynamical rule, which describes the behavior of neurons, does so in a way that shows how the activations of multiple neurons map onto the activation of a new neuron's firing rate, and how the weights of the neurons strengthen the synaptic connections between the new activated neuron and those that activated it.
Hopfield would use McCulloch—Pitts's dynamical rule in order to show how retrieval is possible in the Hopfield network. However, it is important to note that Hopfield would do so in a repetitious fashion. Hopfield would use a nonlinear activation function, instead of using a linear function. This would therefore create the Hopfield dynamical rule and with this, Hopfield was able to show that with the nonlinear activation function, the dynamical master thesis ad hoc networks will always modify the values of the state vector in the direction of one of the stored patterns.
From Wikipedia, the free encyclopedia. Proceedings of the National Academy of Sciences. Bibcode : PNAS doi : PMC PMID Mathematical Biosciences. Reviews of Modern Physics. Bibcode : RvMP Modeling Brain Function: The World of Attractor Neural Networks. Cambridge University Press. ISBN Cerebral Cortex: Principles of Operation.
Oxford University Press. Hopfield Networks". Information Theory, Inference and Learning Algorithms. This convergence proof depends crucially on the fact that the Hopfield network's connections are symmetric.
Ad Hoc Networks
, time: 5:43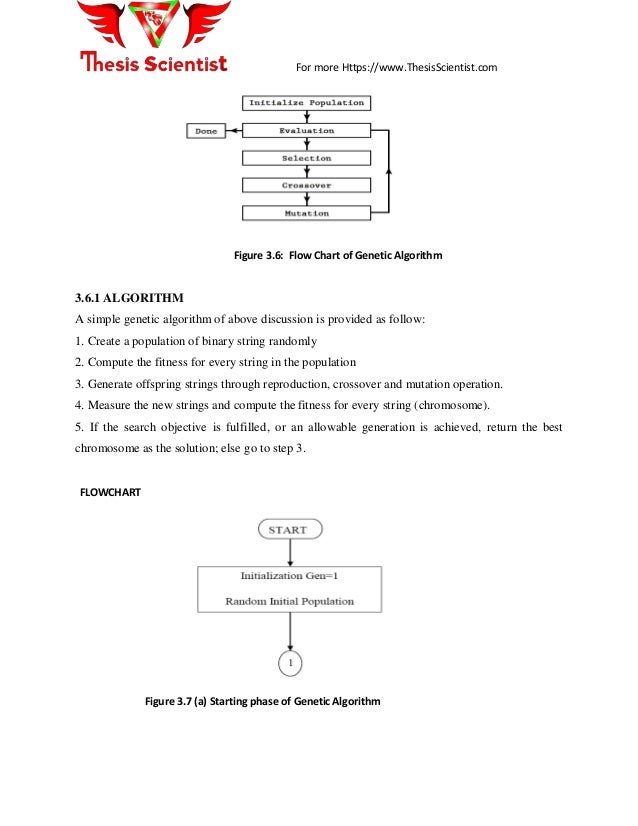
A Hopfield network (or Ising model of a neural network or Ising–Lenz–Little model) is a form of recurrent artificial neural network and a type of spin glass system popularised by John Hopfield in as described earlier by Little in based on Ernst Ising's work with Wilhelm Lenz on the Ising model. Hopfield networks serve as content-addressable ("associative") memory systems with This is the first stable release of Apache Hadoop x line. It contains bug fixes, improvements and enhancements since Users are encouraged to read the overview of major changes since For details of bug fixes, improvements, and other enhancements since the previous release, please check release notes and changelog detail the changes since Master of Engineering. The master of engineering (ME) degree is a two-year program of advanced courses with research leading to a master’s thesis. ME candidates must complete 16 courses (64 credits), including 8 research-oriented courses at the level, complete a thesis
No comments:
Post a Comment