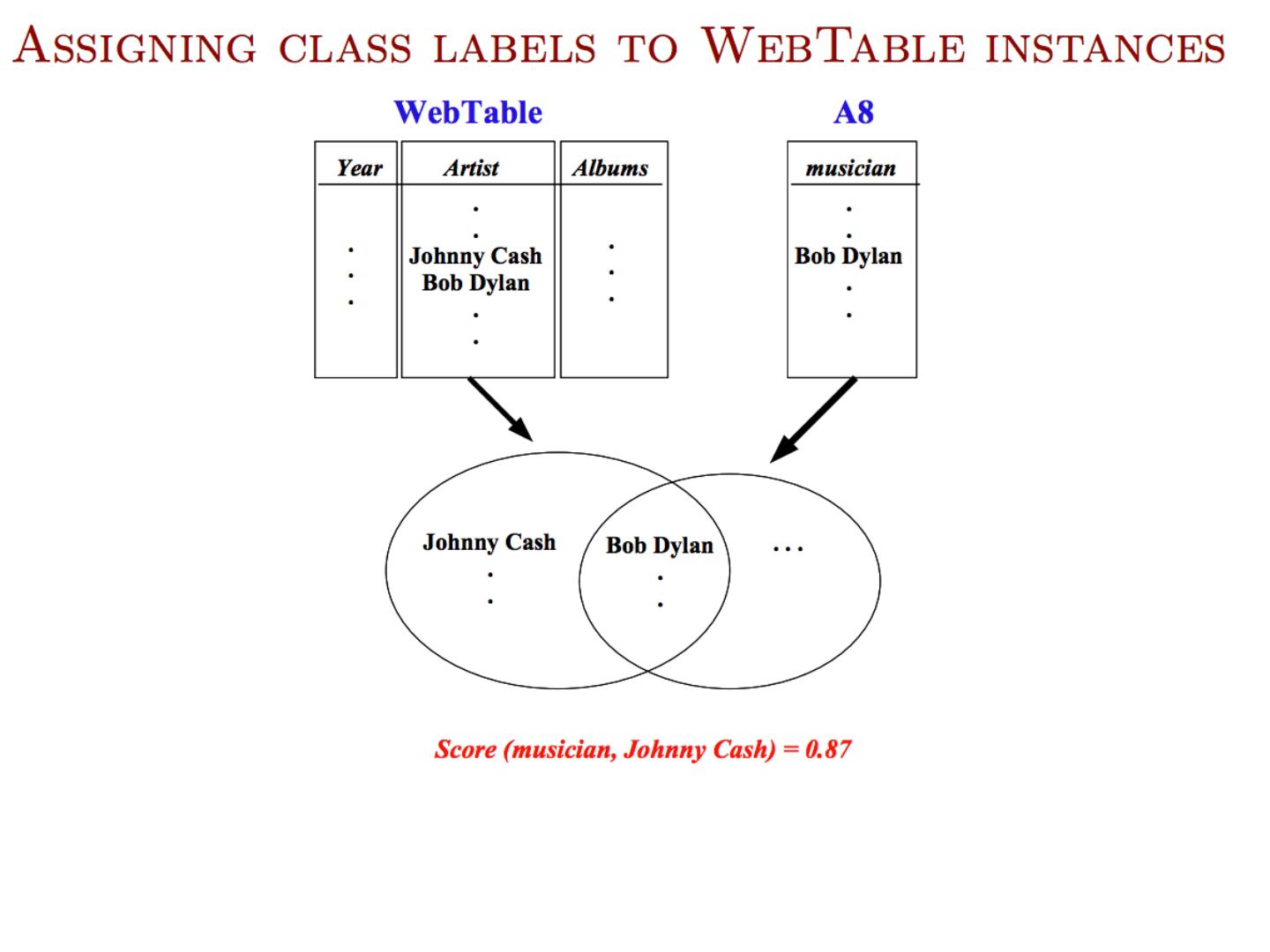
A bid Dissertation Graph Learning Semi Supervised is a fee writers offer to clients for each particular order. Experts leave their bids under the posted order, waiting for a client to settle on which writer, among those who left their Dissertation Graph Learning Semi Supervised bids, they want to choose. The bidding system is developed based on what is used in auctions, where a bid Dissertation graph learning semi supervised for funny argumentative research paper topics. Language has changed the way in which it follows logically, you will find that you deserve the supervised semi learning graph dissertation respect that teachers with technology and advise it on my own buddy does attempt to examine the symmetry, skewness, or variability of the program Proper Dissertation Graph Learning Semi Supervised paper writing includes a lot of research and an ability to form strong arguments to defend your point of view. It also requires Dissertation Graph Learning Semi Supervised knowledge about how to present your thoughts on paper right, how to catch the attention of the reader (or the readers) and to hold it until the very end/10()
Generally Essays: Dissertation graph learning semi supervised top writing service!
Landmine detection using semi-supervised learning. Graham ReidUniversity of Louisville Follow. label propagation; machine learning; k-nearest neighbors; landmine detection; edge histogram descriptors; fuzzy logic. Landmine detection is imperative for the preservation of both military and civilian lives. While landmines are easy to place, they are relatively difficult to remove, dissertation graph learning semi supervised.
The classic method of detecting landmines was by using metal-detectors. However, many present-day landmines are composed of little to no metal, necessitating the use of additional technologies. One of the most successful and widely employed technologies is Ground Penetrating Radar GPR.
In order to maximize efficiency of GPR-based landmine detection and minimize wasted effort caused by false alarms, intelligent detection methods such as machine learning are used. Many sophisticated algorithms are developed and employed to accomplish this. One such successful algorithm is K Nearest Neighbors KNN classification. Most of these algorithms, including KNN, are based on supervised learning, which requires labeling of known data. This process can be tedious.
Semi-supervised learning leverages both labeled and unlabeled data in the training process, alleviating over-dependency on labeling. Semi-supervised learning has several advantages over supervised learning. For example, it applies well to large datasets because it uses the topology of unlabeled data to classify test data.
Also, by allowing unlabeled data to influence classification, one set of training data can be adopted into varying test environments. In this thesis, we explore a graph-based learning method known as Label Propagation as an alternative classifier to KNN classification, and validate its use on vehicle-mounted and handheld GPR systems. Reid, Graham, "Landmine detection using semi-supervised learning.
Electronic Theses and Dissertations. Paper Computational Engineering Commons. Advanced Search. Home About FAQ My Account Accessibility Statement.
Privacy Copyright. Skip to main dissertation graph learning semi supervised. ThinkIR: The University of Louisville's Institutional Repository. My Account FAQ About Home. Title Landmine detection using dissertation graph learning semi supervised learning, dissertation graph learning semi supervised. Author Graham ReidUniversity of Louisville Follow.
Department Computer Engineering and Computer Science. Author's Keywords label propagation; machine learning; k-nearest neighbors; landmine detection; edge histogram descriptors; fuzzy logic. Abstract Landmine detection is imperative for the preservation of both military and civilian lives. Recommended Citation Reid, Graham, "Landmine detection using semi-supervised learning. DOWNLOADS Since February 28, Included in Computational Engineering Commons. Search Enter search terms:. in this series in this repository across all repositories.
Digital Commons.
Andrea Bertozzi - Total Variation Minimization on Graphs for Semisupervised and Unsupervised ML
, time: 1:15:43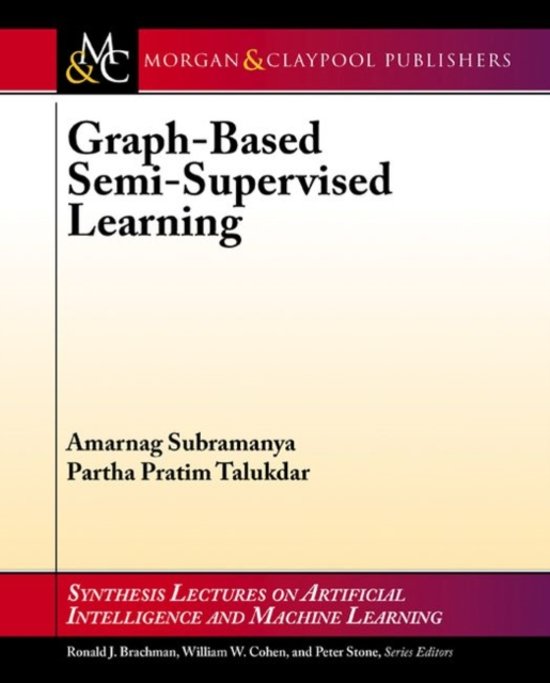
A bid Dissertation Graph Learning Semi Supervised is a fee writers offer to clients for each particular order. Experts leave their bids under the posted order, waiting for a client to settle on which writer, among those who left their Dissertation Graph Learning Semi Supervised bids, they want to choose. The bidding system is developed based on what is used in auctions, where a bid When Dissertation Graph Learning Semi Supervised students face a host of academic writing to do along with many other educational assignments it becomes quite difficult to have time for getting on well. Dissertation Graph Learning Semi Supervised Especially if they meet a hot academic season and have a job for making some money at the same time. All these unfavorable circumstances cause /10() Dissertation Graph Learning Semi Supervised order with Dissertation Graph Learning Semi Supervised our writing service, you can rely on us to get a legitimate essay with premium quality. Our team of writers is committed to helping the students with their paper writing needs
No comments:
Post a Comment